In a world increasingly captivated and transformed by artificial intelligence, the roles of Generative AI and Explainable AI have become pivotal. Yet, their true potential is unleashed only when these two powerful forces intersect. We aim to explore this crucial interplay, diving into how the transparency and understanding provided by Explainable AI are fundamental to harnessing the innovative power of Generative AI.
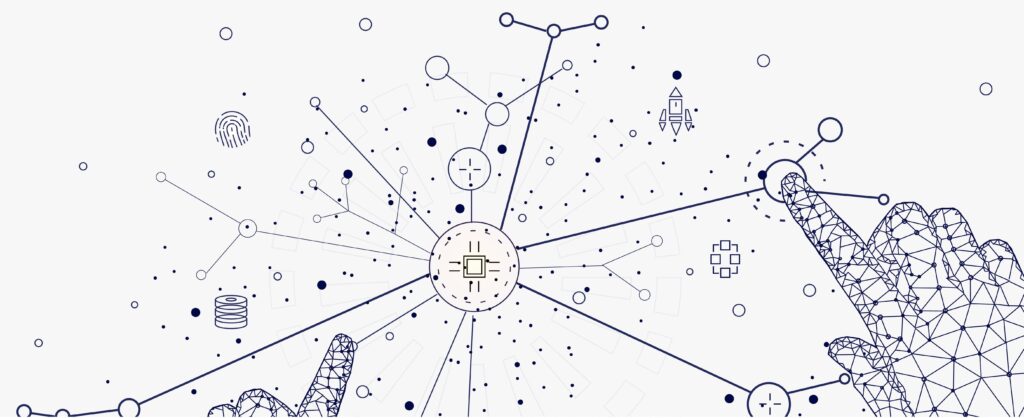
Generative AI, known for its ability to create and innovate, has been making waves across various industries, from digital art to predictive modeling. It represents a frontier of AI that goes beyond analysis and into the realm of creation, producing everything from realistic images and sounds to complex problem-solving strategies. However, the awe-inspiring capabilities of Generative AI come with a need for clarity and trust – a need that Explainable AI fulfills.
Explainable AI, or XAI, serves as the beacon of transparency in the often murky waters of AI operations. It enables us to decipher the ‘how’ and ‘why’ behind AI decisions, making these intelligent systems more understandable and trustable. This aspect is not just a luxury but a necessity, particularly when AI’s decisions impact critical areas like healthcare, finance, and law.
In this blog, we aim to dissect both these fascinating sides of AI, highlighting how Explainable AI doesn’t just complement but significantly empowers Generative AI. Through this exploration, we’ll unveil the myriad ways in which these technologies are not just co-existing but are deeply interconnected, each amplifying the strengths and mitigating the weaknesses of the other.
As we journey through the layers of AI’s creative and explanatory capacities, our goal is to provide insights into why this combination is essential for the sustainable and ethical progression of AI technologies. Whether you are a professional in the tech field, a student of AI, or simply someone intrigued by the rapid advancements in this area, this exploration will offer a comprehensive understanding of the critical relationship between Generative and Explainable AI and its implications for the future of technology.
Understanding Generative AI
Generative AI represents a frontier in the artificial intelligence landscape, primarily focused on creating new and original content. This innovative technology utilizes algorithms trained to understand patterns and features from vast datasets, enabling them to produce unique outputs across various formats, including text, images, sound, and even code. Key technologies underpinning Generative AI include Generative Adversarial Networks (GANs) and Variational Autoencoders (VAEs), each with unique mechanisms and applications including:
- Art and Design: Generative AI has had a transformative impact on the creative industries. AI-generated artworks, created through understanding and recombining elements of style and content from existing art, are now showcased in galleries worldwide.
- Media and Entertainment: In the entertainment sector, Generative AI is used for creating music, generating realistic computer-generated imagery (CGI), and even scripting. Its ability to analyze and replicate styles makes it a powerful tool for content creation.
- Healthcare: One of the most promising applications is in drug discovery and personalized medicine. Here, Generative AI assists in predicting molecular structures and generating new compounds for pharmaceutical development.
- Data Augmentation: Generative models are invaluable for creating synthetic datasets, especially in scenarios where real data is limited or sensitive. This capability is crucial in training other AI models without compromising data privacy.
- Large Language Models (LLMs): Among the most advanced applications of Generative AI are LLMs like OpenAI’s GPT. These models have revolutionized natural language processing (NLP), offering capabilities ranging from generating coherent and contextually relevant text to understanding and translating languages. LLMs serve as a key example of Generative AI’s potential in handling and creating human-like text, making them instrumental in areas like content creation, customer service, and even coding.
Challenges and Limitations
While Generative AI’s potential is immense, there are quite a few challenges:
- Quality and Control: Ensuring high-quality outputs and maintaining control over the nature of the generated content remain ongoing challenges, particularly in preventing the generation of harmful or unethical content.
- Computational Resources: High-end Generative AI models, especially sophisticated LLMs, require substantial computational power, which can be a limiting factor for widespread adoption.
- Data Bias: Models can only be as unbiased as the data they are trained on. Biased training data can lead to skewed or unfair outcomes in the generated content.
- Ethical and Societal Concerns: The potential for misuse, such as creating deepfakes or spreading misinformation through seemingly authentic AI-generated content, raises serious ethical and societal concerns.
- Complexity and Transparency: The intricacy of Generative AI models, particularly LLMs, exemplifies the complexity within this field. Their often opaque nature, where the decision-making process isn’t transparent, underscores the critical need for Explainable AI to demystify these advanced technologies, ensuring responsible and ethical usage.
In summary, Generative AI, with its wide-ranging applications including LLMs, is redefining the boundaries of technological creativity and innovation. However, its rapid advancement brings to the forefront the need for transparency, ethical considerations, and responsible use, areas where Explainable AI can play a vital role.
Exploring Explainable AI
Explainable AI (XAI) refers to the methods and techniques in artificial intelligence that make the results of AI models understandable by humans. Unlike traditional AI models, where the decision-making process can be opaque and difficult to interpret, XAI focuses on transparency and interpretability. This approach allows users and stakeholders to comprehend and trust the decisions made by AI systems.
Importance in the AI Ecosystem
- Trust and Reliability: In critical sectors like healthcare, finance, and law, understanding how AI arrives at a decision is crucial for trust and reliability. XAI helps bridge the gap between AI capabilities and human understanding.
- Compliance and Regulation: As AI becomes more integrated into societal infrastructures, complying with regulations and standards, such as GDPR, which includes the right to explanation, becomes imperative. XAI aids in meeting these regulatory requirements by providing clear insights into AI decisions.
- Bias Detection and Mitigation: XAI plays a key role in identifying and mitigating biases in AI systems. By making the decision-making process transparent, it allows for the examination and correction of underlying biases in data and models.
Techniques and Approaches
- Model Interpretability: This involves designing AI models in a way that their internal mechanics are inherently understandable. Simpler models like decision trees or linear regression naturally lend themselves to this approach.
- Post-hoc Explanation: For more complex models like neural networks, post-hoc techniques provide explanations after the model has made a decision. This could potentially include methods like LIME (Local Interpretable Model-agnostic Explanations) and SHAP (SHapley Additive exPlanations), which help in breaking down and illustrating how a model arrived at a particular outcome.
- Visualization Tools: Visualization is a powerful tool for XAI, providing a more intuitive understanding of complex AI processes. This includes heat maps, graphs, and other visual aids that illustrate the features influencing AI decisions.
Some Applications:
- Healthcare Diagnostics: In healthcare, XAI can elucidate the reasoning behind diagnoses made by AI, aiding doctors in understanding and trusting AI-driven insights.
- Financial Services: In finance, XAI can help in explaining credit scoring models or investment strategies suggested by AI, enhancing transparency and customer trust.
- Legal and Ethical Oversight: In legal contexts, XAI assists in auditing and reviewing decisions made by AI systems, ensuring they comply with legal standards and ethical considerations.
Challenges:
- Complexity vs. Explainability Trade-off: Often, there is a trade-off between the complexity (and hence, the performance) of an AI model and its explainability. The more complex a model, like a deep neural network, the harder it is to make it explainable.
- Subjectivity of Explanations: What constitutes a “good” explanation can be subjective and vary depending on the audience. Tailoring explanations to different stakeholders remains a challenge.
- Computational Overhead: Implementing explainability can add computational overhead to AI systems, potentially affecting performance and efficiency.
Explainable AI is essential in the contemporary AI landscape, ensuring that as AI systems become more advanced and integrated into critical sectors of society, they remain transparent, trustworthy, and aligned with human values and ethics. As AI technologies like Generative AI continue to advance, the role of XAI becomes increasingly important, providing a crucial balance between technological innovation and societal responsibility.
The Symbiosis of Generative and Explainable AI
As the domains of Generative AI and Explainable AI (XAI) evolve, their convergence is not just beneficial but necessary. Generative AI pushes the boundaries of creativity and innovation in AI, while XAI ensures that these advancements are transparent and understandable. This symbiotic relationship is key to harnessing the full potential of AI technologies in a responsible and ethical manner.
Enhancing Trust in Generative Models
- Demystifying Complex Outputs: Generative AI can produce highly sophisticated and intricate outputs, especially in fields like art, content creation, and predictive modeling. XAI tools and techniques make these complex outputs more interpretable and trustworthy for users, who can better understand the ‘how’ and ‘why’ behind AI-generated content.
- Building User Confidence: For AI solutions to be adopted broadly, especially in sectors where decision-making is critical, stakeholders need confidence in the AI’s functionality. XAI provides the necessary transparency to build this confidence, particularly when dealing with sophisticated Generative AI models like Large Language Models (LLMs).
Facilitating Ethical and Responsible AI Use
- Bias Identification and Correction: Generative AI systems, like all AI technologies, can inadvertently perpetuate biases present in their training data. XAI plays a crucial role in identifying these biases by making the model’s decision-making process transparent. This identification is the first step in correcting biases and ensuring fair and ethical use of Generative AI.
- Compliance with Legal and Ethical Standards: As AI becomes more prevalent in sensitive and regulated industries, ensuring compliance with legal and ethical standards is paramount. XAI provides the framework for such compliance, offering clear insights into AI processes and decisions.
Improving AI Development and Deployment
- Iterative Refinement: By understanding how Generative AI models arrive at certain outputs, developers can iteratively refine these models for better accuracy and efficiency. XAI’s insights guide developers in fine-tuning the models, leading to more effective and reliable AI solutions.
- Cross-disciplinary Collaboration: The interaction between Generative AI and XAI fosters a cross-disciplinary approach, combining expertise from data science, psychology, and cognitive science. This collaboration leads to more holistic and human-centric AI systems.
Challenges and Future Directions
- Balancing Performance and Transparency: One of the ongoing challenges is balancing the high performance of Generative AI models with the need for transparency and explainability. Striking this balance is crucial for the wider acceptance and ethical use of AI.
- Developing Standards and Frameworks: There is a growing need for standardized frameworks and guidelines that govern the integration of XAI in Generative AI applications. This would ensure a consistent approach to achieving transparency and explainability across various AI applications.
The interplay between Generative and Explainable AI represents a pivotal aspect of modern AI development. This symbiosis is fundamental to advancing AI technologies in a way that is innovative, trustworthy, and aligned with human values and ethical standards. As both fields continue to evolve, their integration will undoubtedly be a focal point in the journey towards responsible and advanced AI systems.
Ethical Considerations in the Integration of Generative and Explainable AI
The integration of Generative AI with Explainable AI (XAI) brings forth a myriad of ethical considerations. While this synergy promises enhanced transparency and trust in AI systems, it also raises important questions about responsibility, bias, privacy, and the future of human-AI interaction. Addressing these ethical considerations is vital to ensure that the development and deployment of these technologies align with societal values and norms.
Responsibility and Accountability
- Clarifying the AI Decision Chain: As AI systems become more complex, pinpointing responsibility for decisions made by these systems becomes challenging. XAI assists in unraveling this complexity by making the decision-making process more transparent.
- Accountability in AI-driven Outcomes: With Generative AI capable of producing highly autonomous outputs, integrating XAI helps in establishing clear lines of accountability, ensuring that there is a human element accountable for AI decisions.
Bias and Fairness
- Detecting and Mitigating Bias: Both Generative AI and XAI are susceptible to biases inherent in their training data. Ethical integration necessitates rigorous processes to identify and mitigate these biases, ensuring that AI outputs are fair and unbiased.
- Cultural and Contextual Sensitivity: Ensuring that AI systems are sensitive to cultural and contextual nuances is crucial. This involves training models on diverse datasets and using XAI to understand and rectify any culturally insensitive biases.
Privacy Concerns
- Protecting Sensitive Data: Generative AI often requires access to large volumes of data, including potentially sensitive information. Employing XAI must be done with privacy considerations in mind, ensuring that the explanatory processes do not inadvertently expose private data.
- Consent and Transparency in Data Usage: It is essential to obtain informed consent from individuals whose data is used to train or operate these AI systems. Transparency about how data is used, and for what purposes, is a key ethical responsibility.
Impact on Human Skills and Labor
- Complementing vs. Replacing Human Roles: There is a concern that AI, particularly advanced Generative AI, might replace human roles, leading to job displacement. It is important to focus on how AI can complement and augment human skills, rather than simply replacing them.
- Skills Development and Education: As AI takes on more complex tasks, there is a need for upskilling and educating the workforce to work effectively with AI. This includes understanding AI outputs and the use of XAI to make informed decisions.
Future of Human-AI Interaction
- Maintaining Human Oversight: As AI systems become more autonomous, maintaining a level of human oversight is essential to ensure that AI decisions align with ethical and societal norms.
- Fostering Trust and Understanding: The role of XAI is critical in fostering trust and understanding in AI systems, ensuring that people can rely on AI decisions and understand the rationale behind them.
The ethical integration of Generative AI with Explainable AI is not just a technical challenge but a societal imperative. Addressing these ethical considerations ensures that these advanced technologies are developed and used in a manner that is responsible, fair, and aligned with human values. This approach not only enhances the trustworthiness and acceptance of AI technologies but also steers them towards contributing positively to society.
As someone deeply ingrained in Zighra’s journey through the realms of Generative AI models and Explainable AI (XAI), I find myself often reflecting on our approach to solving problems. Our strategy, deeply rooted in leveraging XAI, is not just a business choice; it’s core to our commitment to ethical and transparent AI development.
Being part of the team that integrates XAI into our AI systems, I’ve seen firsthand how vital transparency and understandability are in fostering user trust. It’s not just about building sophisticated systems; it’s about creating an AI that people can believe in and rely upon.
At Zighra, we’ve always believed that user trust is paramount. Our focus on making AI explainable and user-friendly is not just a feature – it’s a core philosophy that drives our innovation. As we continue on our journey at Zighra, the path ahead is clear and exciting. We are not just creating technology; we are shaping a future where AI is seamlessly integrated into the fabric of everyday life, with ethical responsibility and transparency at its core.